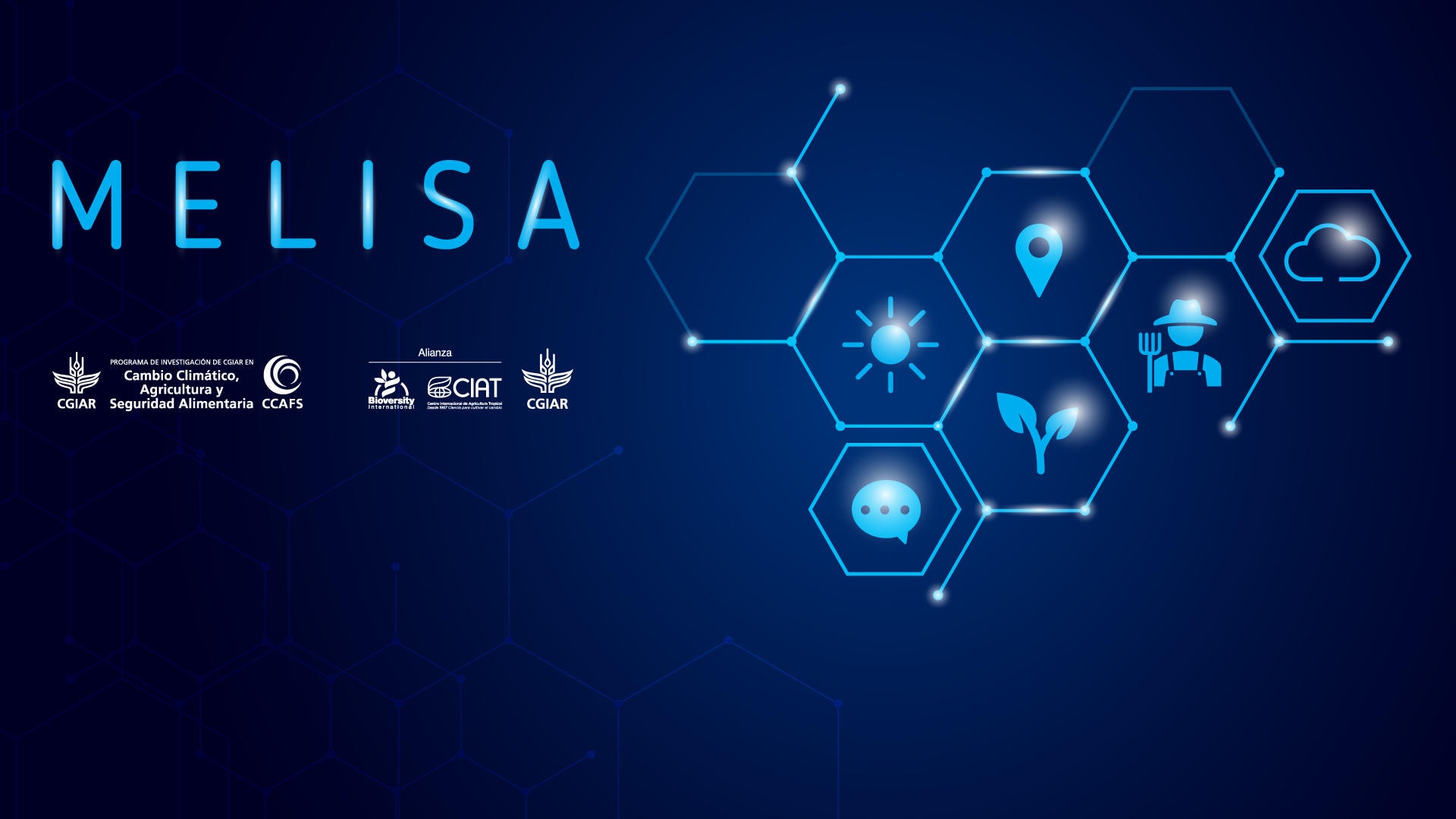
There are some fundamental differences between machine learning and deep-learning. The first relies on unsupervised learn, while the second uses large data sets and powerful computing tools. Let's examine the differences between these two methods and the key difference between them. It helps to have an understanding of the concepts in both. Read this article for more information! We'll also discuss the benefits and drawbacks of each method.
Unsupervised learning
In contrast to supervised learning, which uses data tagged by humans, unsupervised learning relies on a data set without any tags. For example, unsupervised learning algorithms can identify natural clusters or groups based on a dataset. These algorithms, also known as "clustering", can detect correlations between data objects. Unsupervised learning also has an important function: anomaly detection. This is used in banking systems for identifying fraudulent transactions. Unsupervised learning techniques are becoming more common as people attempt to make computers smarter, better able to complete tasks.
There are many problem types that one approach may be more appropriate than the other. This is where supervision and unsupervised methods differ. If reference points and ground reality are not available, supervised learning is ideal. It's not always easy for people to access clean and clearly labeled data. Supervised learning algorithms are best suited to solving real world computation problems. However, unsupervised learning methods can be used to find interesting patterns in data.
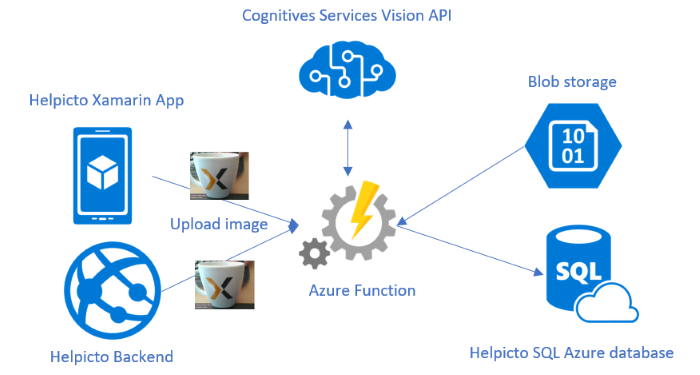
Large data sets
Machine learning uses a variety of data types. There are four main types of data that can be used in machine learning. Each type is based on the task being performed. This article will explain the different types of data that are used in machine-learning and how they can be used to help you create a better machine-learning model. This article also describes some of the most popular ways to extract machine learning data. Here are some of most used methods for extracting machine learning information.
You can find tutorials online to help you get access to large datasets. Kaggle is a community-driven platform that hosts tutorials for hundreds of real-world ML problems. These datasets are typically free and provided by companies, international organizations, and educational institutions like Harvard and Statista. Another source of free data is the Registry of Open Data on AWS, which allows anyone to post datasets. Once you have the data, you will be able to use Amazon data analytics tools for analysis and to make it useful.
Power requirements
The ideal solution for mobile platforms is devices that have AI capabilities. They won't require much power in the near future. The power requirements of these systems are unknown. The cloud providers are not required to disclose their total power consumption for machine-learning systems. Google, Amazon and Microsoft did not comment on the issue. AI systems are an exciting new technology but the current power requirements are not sustainable.
As training datasets grow, so do the power requirements of machine learning algorithms. A single V100 GPU consumes about 250-300 watts. A system with 128,000 watts (or 128 kilowatts) of V100 GPUs would consume 128,000 W. MegatronLMs were used in one study to train a neural networks. The system consumed 27,648kWh. That's about the same energy as three houses. New methods of training machine learning algorithms are being developed to reduce their energy consumption. However, many models still require enormous data to train.
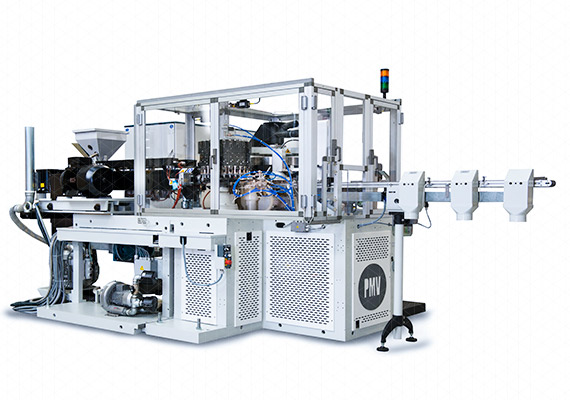
Applications
As a powerful tool for business intelligence, analytics vendors, and resource management systems, machine learning and deep learning are used in a variety of applications. Semi-autonomous cars use machine learning algorithms to recognize partially visible objects. And a smart assistant usually combines supervised and unsupervised machine learning models to interpret natural speech and provide context. These methods are growing in popularity. Continue reading to learn more about machine learning and deeplearning.
Facebook, one of the most popular social networking platforms, uses machine-learning algorithms to automatically classify photos. Facebook creates albums containing photos tagged with users and automatically labels uploaded pictures. Google Photos however uses deep learning for every element in a photo. One of the most striking examples of Deep Learning applications is product recommendations. E-commerce websites use this technique to track user behavior and make product recommendations based on past purchases. This technology can be used for example in a smart face lock.
FAQ
How will governments regulate AI
The government is already trying to regulate AI but it needs to be done better. They must ensure that individuals have control over how their data is used. Companies shouldn't use AI to obstruct their rights.
They must also ensure that there is no unfair competition between types of businesses. For example, if you're a small business owner who wants to use AI to help run your business, then you should be allowed to do that without facing restrictions from other big businesses.
How does AI impact the workplace
It will transform the way that we work. It will allow us to automate repetitive tasks and allow employees to concentrate on higher-value activities.
It will improve customer service and help businesses deliver better products and services.
It will enable us to forecast future trends and identify opportunities.
It will allow organizations to gain a competitive advantage over their competitors.
Companies that fail AI adoption will be left behind.
How does AI work?
An artificial neural networks is made up many simple processors called neuron. Each neuron receives inputs form other neurons and uses mathematical operations to interpret them.
Neurons can be arranged in layers. Each layer serves a different purpose. The first layer gets raw data such as images, sounds, etc. These are then passed on to the next layer which further processes them. The final layer then produces an output.
Each neuron has an associated weighting value. This value is multiplied with new inputs and added to the total weighted sum of all prior values. If the result is more than zero, the neuron fires. It sends a signal along the line to the next neurons telling them what they should do.
This cycle continues until the network ends, at which point the final results can be produced.
What industries use AI the most?
The automotive sector is among the first to adopt AI. BMW AG uses AI for diagnosing car problems, Ford Motor Company uses AI for self-driving vehicles, and General Motors uses AI in order to power its autonomous vehicle fleet.
Other AI industries include banking and insurance, healthcare, retail, telecommunications and transportation, as well as utilities.
Where did AI get its start?
In 1950, Alan Turing proposed a test to determine if intelligent machines could be created. He suggested that machines would be considered intelligent if they could fool people into believing they were speaking to another human.
John McCarthy, who later wrote an essay entitled "Can Machines Thought?" on this topic, took up the idea. In 1956, McCarthy wrote an essay titled "Can Machines Think?" He described the problems facing AI researchers in this book and suggested possible solutions.
What is AI and why is it important?
According to estimates, the number of connected devices will reach trillions within 30 years. These devices will include everything from cars to fridges. Internet of Things (IoT), which is the result of the interaction of billions of devices and internet, is what it all looks like. IoT devices and the internet will communicate with one another, sharing information. They will be able make their own decisions. A fridge might decide whether to order additional milk based on past patterns.
It is estimated that 50 billion IoT devices will exist by 2025. This is an enormous opportunity for businesses. It also raises concerns about privacy and security.
Is Alexa an AI?
The answer is yes. But not quite yet.
Amazon's Alexa voice service is cloud-based. It allows users to interact with devices using their voice.
The technology behind Alexa was first released as part of the Echo smart speaker. However, since then, other companies have used similar technologies to create their own versions of Alexa.
Some of these include Google Home, Apple's Siri, and Microsoft's Cortana.
Statistics
- A 2021 Pew Research survey revealed that 37 percent of respondents who are more concerned than excited about AI had concerns including job loss, privacy, and AI's potential to “surpass human skills.” (builtin.com)
- The company's AI team trained an image recognition model to 85 percent accuracy using billions of public Instagram photos tagged with hashtags. (builtin.com)
- In the first half of 2017, the company discovered and banned 300,000 terrorist-linked accounts, 95 percent of which were found by non-human, artificially intelligent machines. (builtin.com)
- That's as many of us that have been in that AI space would say, it's about 70 or 80 percent of the work. (finra.org)
- More than 70 percent of users claim they book trips on their phones, review travel tips, and research local landmarks and restaurants. (builtin.com)
External Links
How To
How to set Cortana up daily briefing
Cortana in Windows 10 is a digital assistant. It is designed to help users find answers quickly, keep them informed, and get things done across their devices.
To make your daily life easier, you can set up a daily summary to provide you with relevant information at any moment. The information should include news, weather forecasts, sports scores, stock prices, traffic reports, reminders, etc. You have the option to choose which information you wish to receive and how frequently.
Win + I will open Cortana. Scroll down to the bottom until you find the option to disable or enable the daily briefing feature.
Here's how you can customize the daily briefing feature if you have enabled it.
1. Open Cortana.
2. Scroll down to "My Day" section.
3. Click the arrow to the right of "Customize My Day".
4. You can choose which type of information that you wish to receive every day.
5. You can change the frequency of updates.
6. Add or remove items from your shopping list.
7. Save the changes.
8. Close the app